What Is Unstructured Data in AI?
- learnwith ai
- Apr 7
- 3 min read
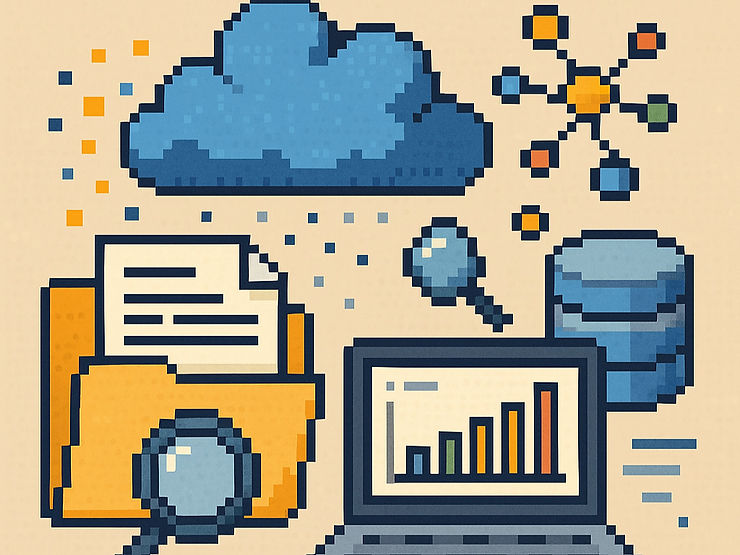
Artificial Intelligence thrives on data. Yet, not all data is created equal. While structured data fits neatly into databases and spreadsheets, unstructured data emails, images, videos, social media posts, PDFs, and more makes up over 80 percent of the world’s digital information. For AI systems, this is both a goldmine and a challenge.
What is Unstructured Data?
Unstructured data lacks a predefined format. It cannot be easily stored in rows and columns like traditional databases. Examples include:
Text from customer reviews or support tickets
Audio files and voice recordings
Images and videos
Log files and sensor data
Social media posts and emails
This kind of data is rich with context and meaning, but machines cannot immediately interpret it without preprocessing or specialized tools.
Why Unstructured Data Matters in AI
Unstructured data holds valuable insights that structured datasets might miss. Consider a healthcare AI that analyzes patient reports. Structured data might include age and diagnosis, but unstructured text can reveal symptoms, lifestyle habits, or emotional tone critical for holistic analysis.
In marketing, analyzing customer feedback from social platforms or emails provides a deeper understanding of brand perception, customer needs, and sentiment.
However, the biggest roadblock is that unstructured data is messy, inconsistent, and hard to analyze using traditional tools.
The Challenges
Volume and Variety: The sheer scale and range of formats make storage and management difficult.
Noise: Unstructured data often includes irrelevant or redundant information.
Interpretability: Natural language, visual patterns, and non-numeric formats require complex models to interpret.
Labeling and Annotation: Supervised learning requires labeled datasets, and labeling unstructured data is time-consuming.
How to Fix It: Turning Chaos into Clarity
Solving the unstructured data problem involves a blend of AI techniques, human oversight, and smart infrastructure. Here’s how:
1. Natural Language Processing (NLP)
NLP allows machines to understand, interpret, and generate human language. Sentiment analysis, topic modeling, and named entity recognition can structure raw text for downstream AI tasks.
2. Computer Vision
For image and video data, computer vision algorithms extract patterns, detect objects, and even interpret emotions. Pretrained models like ResNet or YOLO streamline this process.
3. Speech Recognition and Audio Analysis
Audio can be transcribed using automatic speech recognition (ASR) systems. From there, NLP techniques can analyze the resulting text.
4. Data Labeling Tools and Human-in-the-Loop Systems
Tools like Labelbox, Prodigy, or Snorkel help automate and manage data annotation. A human-in-the-loop approach combines machine suggestions with expert validation, reducing time and improving accuracy.
5. Data Lakes and Scalable Storage
Rather than forcing unstructured data into rigid formats, data lakes allow flexible storage. Metadata tagging and schema-on-read approaches enhance discoverability.
6. AI-Powered ETL Pipelines
Modern extract-transform-load (ETL) tools integrate AI to preprocess and enrich unstructured data, preparing it for model training or analytics.
Real-World Use Cases
Finance: AI extracts key data points from contracts and PDFs for compliance and automation.
Retail: Customer reviews are analyzed to refine product features.
Healthcare: Physician notes are transformed into structured formats for diagnosis support.
Cybersecurity: Log files and threat reports are mined for patterns indicating attacks.
Final Thoughts
Unstructured data is not a flaw in the system it is a feature of the modern digital world. By applying the right combination of AI technologies and data engineering strategies, organizations can unlock its true potential.
The future of AI lies not in perfect data, but in its ability to extract order from complexity.
—The LearnWithAI.com Team