What is Symbolic AI vs. Subsymbolic AI?
- learnwith ai
- 6 days ago
- 3 min read
Updated: 3 days ago
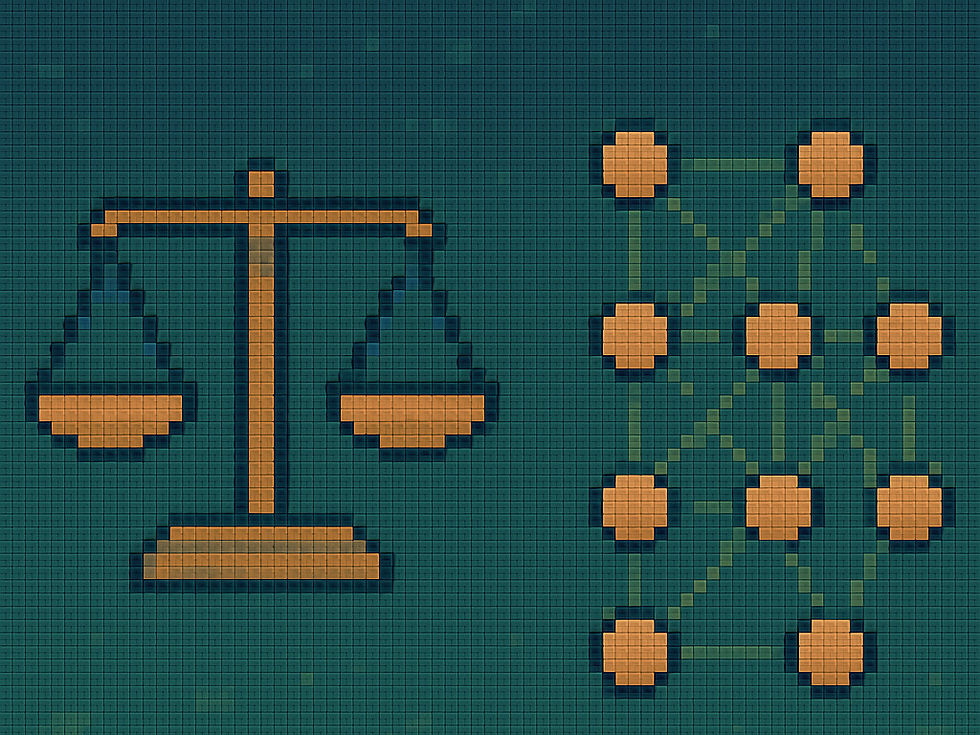
What is Symbolic AI?
Picture Symbolic AI as a master chess player, carefully plotting moves using a rulebook carved in stone. Known as Good Old-Fashioned AI (GOFAI), this approach represents knowledge with symbols think of them as building blocks for objects, ideas, or connections and manipulates them with logical rules. For instance, in a medical system, it might deduce, “If a patient has a fever and a cough, they might have the flu.” This clarity makes Symbolic AI a beacon of transparency, perfect for scenarios where every decision needs a paper trail, like legal rulings or expert diagnostics.
Its strengths shine bright: humans can peek under the hood and follow the logic, step by step. It thrives in structured domains where knowledge can be neatly packaged into “if-then” statements. Yet, it stumbles in murky waters. Uncertainty throws it off balance, and crafting those rules by hand is a Herculean task, often dubbed the “knowledge acquisition bottleneck.” Miss a rule, and the system might freeze, like a chess player stumped by an unexpected move.
What is Subsymbolic AI?
Now, envision Subsymbolic AI as a child learning to spot animals in a picture book, soaking up patterns without a single “why” or “how.” This approach, rooted in connectionist models like artificial neural networks, builds intelligence from countless tiny units working in harmony. Knowledge isn’t written in plain English but woven into the weights linking these neurons, adjusted through floods of data. From recognizing faces in photos to powering chatbots,
Subsymbolic AI dominates tasks that demand intuition over explanation.
Its superpower? It learns from the chaos of real-world data, excelling at spotting intricate patterns and adapting to new challenges. Whether it’s deciphering handwriting or translating languages, it handles noise and complexity with ease. But here’s the catch: it’s a mysterious artist. Peek inside, and you’ll find a “black box,” where decisions emerge without a clear story. Plus, it guzzles data and computing power like a hungry beast, a trade-off for its brilliance.
Symbolic vs. Subsymbolic: The Great Debate
Let’s stage a friendly showdown between these two. Symbolic AI stands tall with its rulebook, offering a crystal-clear map of its reasoning. Subsymbolic AI, meanwhile, dances through data, delivering answers with a flair that’s hard to dissect. Need an AI to justify its choices in a courtroom or hospital? Symbolic takes the crown. Tasked with identifying objects in a blurry video or mastering a game through trial and error? Subsymbolic steals the show.
Consider chess, a perfect battleground. A Symbolic AI might lean on algorithms like minimax, evaluating moves with precise heuristics: “Protect the king, capture the queen.” A Subsymbolic AI, however, might study millions of games, learning to “feel” winning positions without ever touching a rulebook. In practice, champions like AlphaZero blend both, pairing neural intuition with structured search—a hint that these rivals can be allies.
The Future: A Harmony of Minds?
As AI evolves, the lines between Symbolic and Subsymbolic blur. Hybrid systems, like neuro-symbolic AI, marry the logic of rules with the adaptability of networks, aiming for machines that both perform and explain. Subsymbolic AI has fueled jaw-dropping leaps in vision and language, yet the call for transparency grows louder, nudging Symbolic AI back into the spotlight. The future might not be a victor’s tale but a duet, harmonizing precision and instinct for smarter, trustier AI.
So, next time you marvel at an AI’s feat, ask: Is it a librarian or an artist at work? Understanding these paradigms unlocks not just how machines think, but how they can best serve us.
—The LearnWithAI.com Team