What is Multi-task Learning in AI?
- learnwith ai
- Apr 4
- 2 min read
Updated: Apr 5
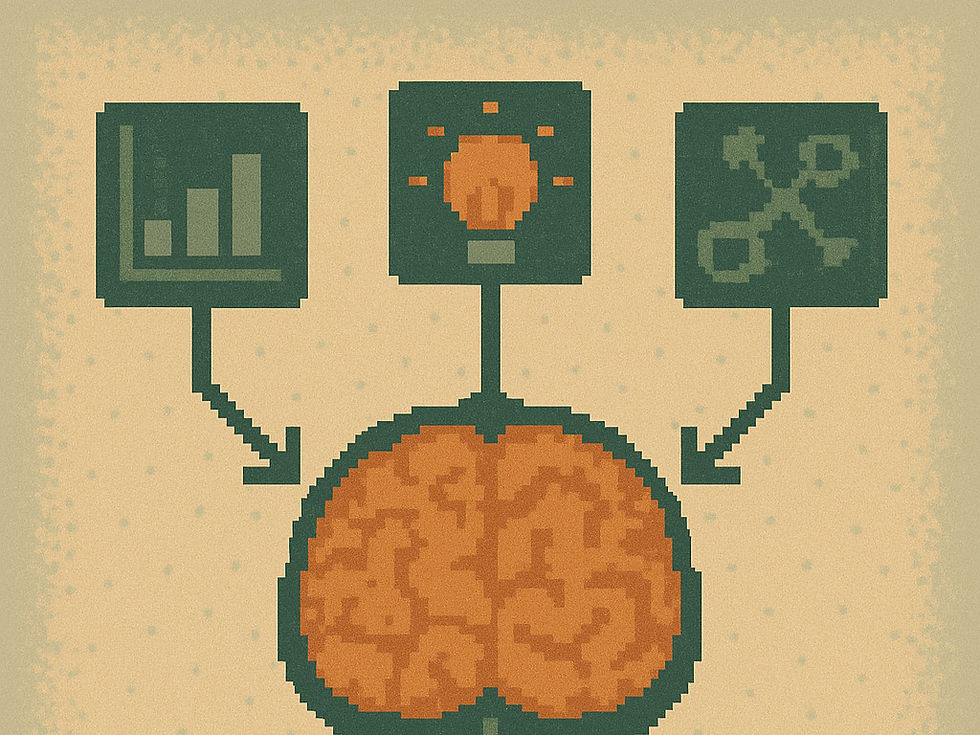
Artificial Intelligence continues to evolve beyond single-purpose models. Today, systems are expected to understand images, translate languages, summarize documents, and even detect fraud—sometimes all in one go.
This is where Multi-task Learning (MTL) comes into play.
Rather than training separate models for each task, Multi-task Learning empowers a single model to learn and perform multiple tasks simultaneously. Think of it like a human who learns to read and write in several languages at once. Each task reinforces the other, leading to better comprehension overall.
Why Multi-task Learning Matters
MTL breaks the mold of narrow AI by promoting more generalizable knowledge. This learning paradigm doesn’t just save computational resources—it boosts accuracy by leveraging shared representations between related tasks. For example, a model learning to recognize handwritten digits can also learn to detect strokes or curves, helping both tasks improve in parallel.
How It Works
At the core of Multi-task Learning lies the idea of shared representations. Instead of building isolated neural networks, MTL architectures often share the initial layers across tasks, with task-specific layers at the end. These shared layers extract common patterns—like edges in images or syntax in language—while the final layers specialize for each output.
This shared learning helps reduce overfitting, especially in smaller datasets. By introducing complementary tasks, the model avoids focusing too narrowly and instead builds a more robust understanding.
Real-World Applications of MTL
Natural Language Processing (NLP):In models like BERT or T5, tasks such as sentiment analysis, question answering, and translation can be learned together.
Computer Vision:Object detection, segmentation, and depth estimation often share visual cues. MTL models unify these into a single process.
Healthcare:Models diagnosing multiple diseases from the same medical imaging data benefit from shared anatomical patterns.
Autonomous Vehicles:A single model may need to detect lanes, recognize traffic signs, and predict pedestrian movement—all at once.
Benefits of Multi-task Learning
Improved Generalization: Learning multiple tasks discourages the model from overfitting on any single one.
Efficiency: MTL reduces the need to train and maintain separate models, cutting down on resource usage.
Knowledge Transfer: Information learned from one task can help another, especially when data is limited.
Scalability: MTL paves the way for more adaptable and multifunctional AI systems.
Challenges to Consider
Task Conflict: Sometimes, tasks may compete or interfere, reducing performance across the board.
Data Imbalance: Some tasks may dominate the training if not balanced properly, skewing the model’s learning.
Architecture Design: Structuring the network to accommodate different tasks without loss of performance is non-trivial.
Conclusion: A Smarter Path to General AI
Multi-task Learning represents a key step toward more intelligent, adaptable, and resource-efficient AI systems. By teaching models to perform multiple functions in tandem, we open the door to truly generalizable learning—mirroring the way humans tackle diverse challenges through interconnected knowledge.
As research continues to evolve, MTL will likely become foundational in the next wave of AI breakthroughs, particularly in building models that can think beyond one problem at a time.
—The LearnWithAI.com Team