What is Metadata in AI?
- learnwith ai
- 6 days ago
- 3 min read
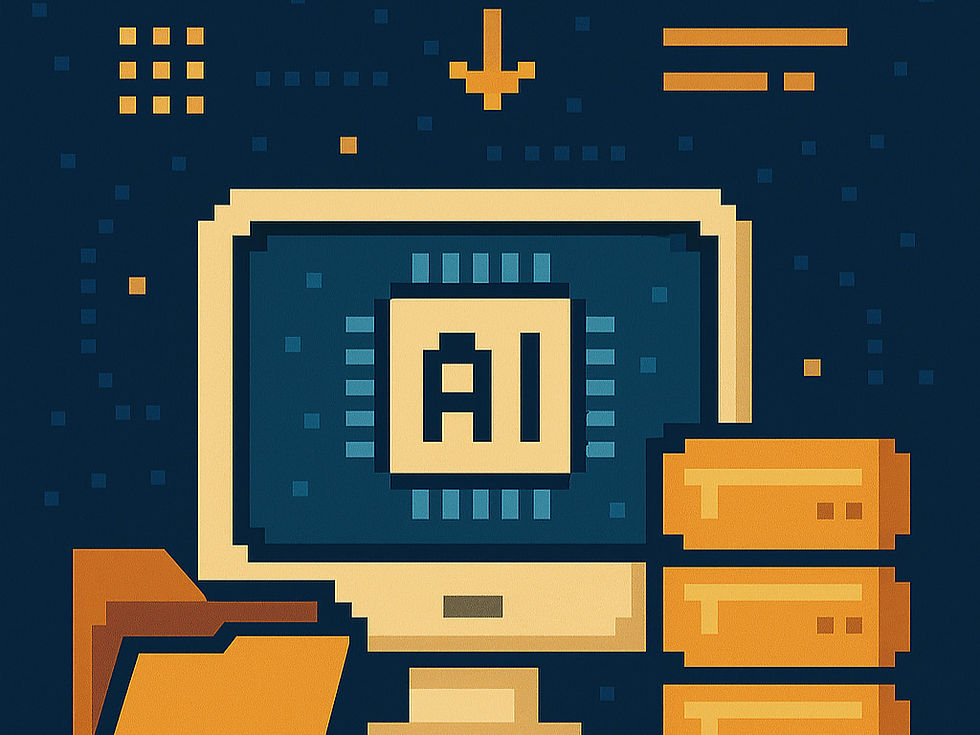
In the fast-evolving world of artificial intelligence, data is everything. But behind every dataset lies a layer of invisible yet powerful information metadata. Often described as “data about data,” metadata plays a foundational role in making AI systems smarter, faster, and more ethical.
Understanding Metadata in Simple Terms
Imagine you have a photograph of a cat. The image itself is data. Now think about the information describing it the date it was taken, the camera settings, the location, or even a label that says “cat.” That descriptive layer is metadata.
In artificial intelligence, metadata does more than just describe. It defines the context, meaning, and structure of the data used to train, test, and deploy machine learning models. Without metadata, AI systems are left in the dark, attempting to make sense of a world without guidance.
Why Metadata Matters in AI
Metadata serves multiple critical functions in AI development:
1. Data Labeling and Classification
Machine learning models rely on labeled data to learn. Metadata provides those labels for instance, identifying whether an image shows a cat, dog, or bird. The more accurate the metadata, the more reliable the model’s predictions.
2. Data Discovery and Retrieval
AI systems often process vast datasets. Metadata acts like a searchlight, enabling fast discovery and retrieval of relevant data based on tags, categories, or parameters.
3. Data Lineage and Governance
Metadata tracks the origin and transformation of data. This is crucial for compliance, reproducibility, and ethical AI practices, as it allows developers to audit how data was collected, processed, and used.
4. Model Training Efficiency
Well-structured metadata helps optimize training pipelines. It enables filtering out irrelevant data, selecting appropriate subsets, and identifying bias or gaps in data distribution.
5. Interoperability and Standardization
In collaborative AI environments, metadata ensures that datasets speak the same language. Standards like JSON-LD or schema.org help harmonize data from different sources.
Types of Metadata in AI Projects
AI systems use a variety of metadata types:
Descriptive Metadata: Tags, labels, keywords
Structural Metadata: Data formats, schemas, hierarchies
Administrative Metadata: Licensing, access controls, data ownership
Provenance Metadata: Origins, changes over time, version histories
Statistical Metadata: Metrics, distributions, outliers
Each type contributes to the integrity and intelligence of AI workflows.
Real-World Applications of Metadata in AI
Healthcare: Labeling X-rays with metadata improves diagnostic AI accuracy
Finance: Time-stamped transaction metadata powers fraud detection systems
Retail: Metadata from customer behavior enhances recommendation engines
Autonomous Vehicles: Metadata from sensor inputs supports object recognition
Metadata and Ethical AI
As AI becomes more autonomous, questions of bias, fairness, and transparency grow louder. Metadata provides the breadcrumbs for accountability. By documenting how data is sourced and labeled, metadata enables external audits and supports explainable AI — making it easier to understand and trust machine decisions.
Best Practices for Managing Metadata in AI
Automate metadata generation using tools integrated with data pipelines
Adopt metadata standards like Dublin Core or ML Metadata (MLMD)
Continuously update metadata as datasets evolve
Visualize metadata to uncover patterns, gaps, or inconsistencies
Secure sensitive metadata, especially in regulated industries
The Future of Metadata in AI
With the rise of data-centric AI development, metadata will only become more critical. Future models will depend not just on big data, but on smart data and smart data begins with rich, meaningful metadata.
As AI systems grow more complex, metadata will act as their memory, their logic, and their moral compass. It is the silent architect behind intelligent decisions.
—The LearnWithAI.com Team