What is Image Data in AI?
- learnwith ai
- Apr 7
- 2 min read
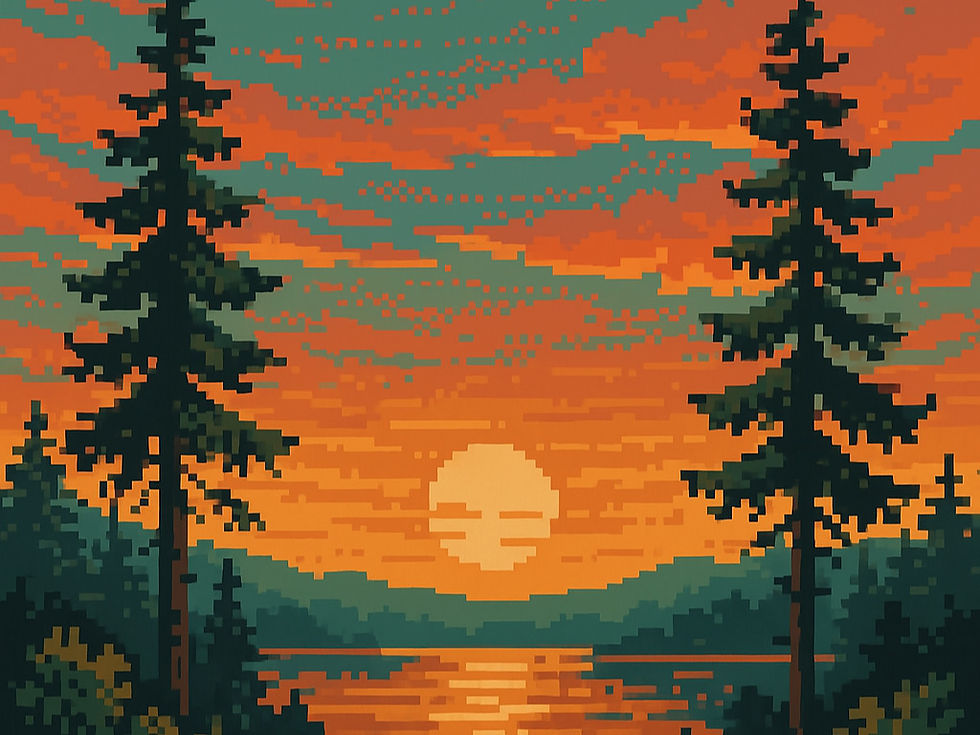
Artificial Intelligence has given machines the ability to hear, read, and even see. Among these capabilities, image understanding stands out as one of the most complex—and transformative. But what exactly is image data in the world of AI, and how do machines make sense of it?
What is Image Data?
At its core, image data refers to the digital representation of visual information. Every image is made up of pixels, each carrying numerical values that define color and brightness. To a computer, a picture of a cat is not a cute animal—it is a matrix filled with numbers.
Depending on the format, an image might be represented as:
A 2D matrix (for grayscale images)
A 3D matrix (for RGB images, including three color channels: Red, Green, Blue)
For example, a 100x100 pixel RGB image would be interpreted as a 100 x 100 x 3 numerical array.
Why is Image Data Important in AI?
Image data fuels computer vision, the field of AI that enables machines to interpret and make decisions based on visual inputs. This technology powers:
Facial recognition in smartphones
Autonomous vehicle navigation
Medical imaging diagnostics
Product detection in retail
Security and surveillance analytics
Without high-quality, well-labeled image data, these systems would not be able to learn patterns or recognize features.
From Pixels to Patterns: How AI Learns from Images
When training an AI model, image data goes through several stages:
Preprocessing – resizing, normalization, or filtering to enhance consistency
Labeling – adding context (e.g., “dog,” “cat,” “car”) for supervised learning
Augmentation – creating variations like flips, rotations, or brightness changes to increase data diversity
Feature Extraction – identifying edges, textures, shapes, and patterns that define objects
Classification/Detection – using learned features to categorize or locate objects
Deep learning models, particularly Convolutional Neural Networks (CNNs), are the most commonly used architectures for analyzing image data. They learn to detect patterns by scanning small areas (filters) of the image, layer by layer.
Challenges with Image Data
While powerful, image data presents unique hurdles:
Size and Storage: Image files can be large, requiring significant processing power and memory.
Labeling Cost: Manual annotation is time-consuming and expensive.
Bias and Quality: Poor data quality or biased datasets can lead to inaccurate or unfair AI decisions.
The Future of Image Data in AI
As AI continues to evolve, so will its capacity to understand visuals. New techniques like self-supervised learning, transformer-based vision models, and synthetic image generation are expanding what’s possible.
Image data is no longer just about recognition—it’s about understanding context, emotion, and even creativity. From AI-generated art to real-time video analytics, the future is being seen through a new lens.
Final Thoughts
Image data is not just a resource it is the visual language machines are learning to speak. As we move deeper into the era of intelligent systems, our understanding of how machines interpret the world will shape everything from how we shop to how we stay safe.
The next time you snap a photo, remember: to AI, it is more than a picture. It is data. And data is power.
—The LearnWithAI.com Team