What is Federated Learning in AI?
- learnwith ai
- 3 days ago
- 2 min read
Updated: 2 days ago
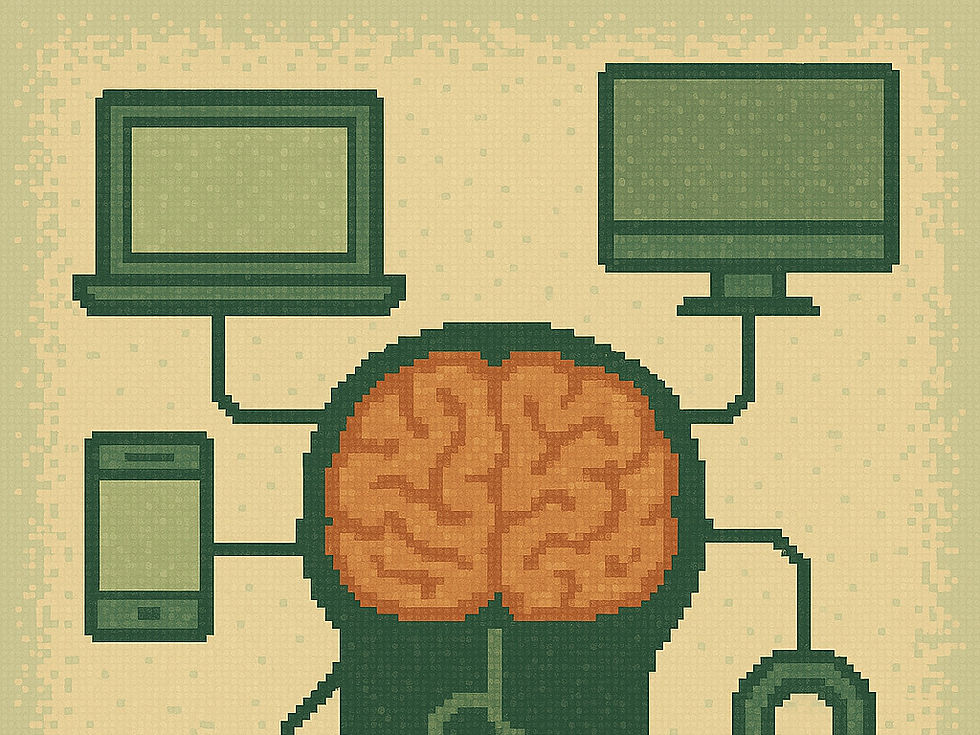
Imagine millions of users with smartphones. Each phone generates data daily—texts, photos, app usage—but none of this data ever leaves the device. Instead of uploading that sensitive data to a cloud for model training, Federated Learning sends a small version of the model to each phone.
Locally, the model learns from user behavior. Then, instead of transmitting raw data, each device sends only the updated model weights back to a central server. These updates are aggregated into a global model, which continuously improves with every round.
This decentralized method respects user privacy while still enabling the AI to learn effectively across a diverse set of data sources.
Key Benefits of Federated Learning
1. Data Privacy at the Core
No personal data ever leaves the device. This method inherently supports data protection and compliance with privacy laws like GDPR or HIPAA.
2. Reduced Latency
Training and inference happen locally, resulting in faster responses—essential for time-sensitive applications like predictive keyboards or voice assistants.
3. Bandwidth Efficiency
Since only model updates are sent (and often compressed), network bandwidth is conserved. That’s a win for scalability.
4. Real-World Relevance
Training occurs in the real world, on real devices, making the resulting models more robust and adaptable to diverse environments.
Use Cases of Federated Learning
Healthcare: Train models on patient data from multiple hospitals without sharing sensitive information.
Finance: Improve fraud detection across banks without exposing client data.
Mobile Applications: Refine keyboard predictions, facial recognition, or health tracking on smartphones in real-time.
Challenges of Federated Learning
Like any innovation, Federated Learning has its limitations. Training on edge devices means managing computational constraints, unreliable connectivity, and heterogeneous data formats. Moreover, ensuring model robustness against adversarial updates requires careful orchestration and security controls.
But these challenges are actively being addressed, with advancements like secure aggregation, differential privacy, and homomorphic encryption offering layers of protection and optimization.
The Future of Federated Intelligence
As data privacy regulations tighten and public awareness grows, Federated Learning is poised to play a central role in AI’s next chapter. It aligns perfectly with the vision of ethical, transparent, and user-centric AI—where intelligence doesn’t come at the cost of privacy.
Tech giants like Google, Apple, and NVIDIA are already investing heavily in this approach. In the coming years, expect Federated Learning to be foundational in applications ranging from autonomous vehicles to smart homes and beyond.
Conclusion
Federated Learning redefines how machines learn—decentralized, private, and scalable. It's not just a technical innovation; it's a philosophical one. In a world where trust is paramount, this paradigm allows AI to evolve without compromising the very people it serves.
—The LearnWithAI.com Team