What is Audio Data in AI?
- learnwith ai
- Apr 7
- 2 min read
Updated: Apr 8
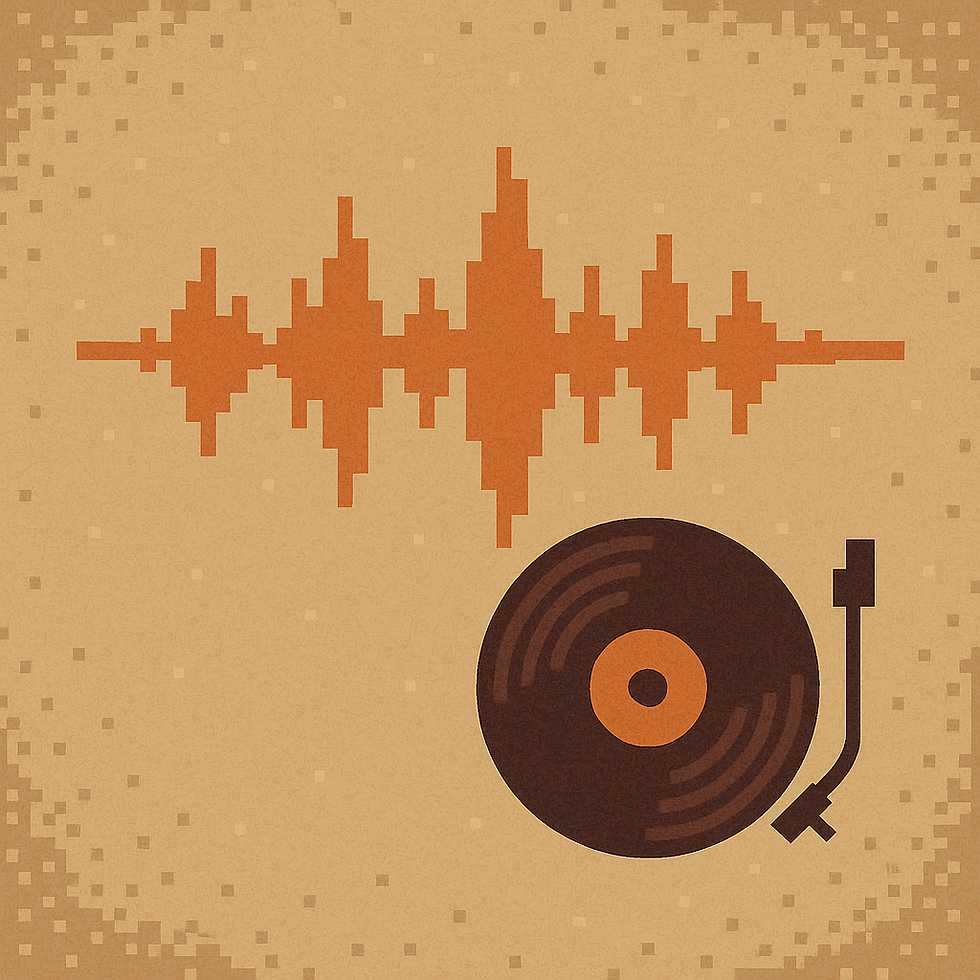
Audio data refers to any sound captured and stored in a format a computer can process. It might include human speech, music, environmental noises, or even inaudible frequencies. The most common formats are WAV, MP3, FLAC, and AAC, but for AI purposes, audio is often transformed into waveforms, spectrograms, or Mel-frequency cepstral coefficients (MFCCs) to be fed into models.
Why is Audio Data Important in AI?
Speech Recognition AI systems use audio data to convert speech into text. This is the core of technologies like voice typing and call transcription.
Voice Assistants Devices like Alexa and Google Assistant rely on audio input to interpret commands and interact with users.
Emotion Detection By analyzing tone, pitch, and rhythm, AI can detect human emotions in real-time conversations.
Music and Sound Classification AI can identify genres, instruments, and even detect copyright violations in audio clips.
Accessibility Tools Audio-driven AI supports the visually impaired through screen readers and voice-based navigation.
How AI Processes Audio
To make sense of sound, AI systems break down audio signals into numeric representations. The key steps include:
Sampling: Capturing the amplitude of a sound wave at intervals.
Fourier Transform: Converting time-based signals into frequency-based data.
Spectrogram Creation: Visualizing frequency over time, often used in deep learning models.
Deep learning models like Convolutional Neural Networks (CNNs) and Recurrent Neural Networks (RNNs) are commonly used to process audio data, especially when transformed into spectrograms.
Audio Data vs Other Types of Data
Unlike tabular or image data, audio is temporal, meaning it unfolds over time. This makes it uniquely suited for sequence modeling. While image data captures a single moment, audio can tell a story—word by word, note by note.
Challenges of Working with Audio Data
Background Noise Environmental sounds can interfere with clarity.
Accents and Dialects Diverse speech patterns challenge models trained on limited datasets.
Data Volume High-quality audio requires significant storage and processing power.
Labeling Complexity Annotating audio data for machine learning can be time-intensive and subjective.
The Future of Audio in AI
Advancements in natural language understanding and generative models are taking audio analysis to new heights. AI is now capable of generating lifelike voices, translating speech across languages, and even composing music. As edge devices become more powerful, real-time audio processing is becoming more accessible, enabling smarter homes, cars, and wearable tech.
Conclusion
Audio data is more than just sound—it’s a rich, multi-dimensional input that allows machines to understand, interact, and respond in profoundly human ways. Whether guiding us through a map or responding to our voice in a smart speaker, audio fuels some of the most seamless AI experiences in our daily lives. Mastering this type of data is essential for building truly intelligent systems.
—The LearnWithAI.com Team