What is a Dataset in AI?
- learnwith ai
- Mar 28
- 4 min read
Updated: 2 days ago
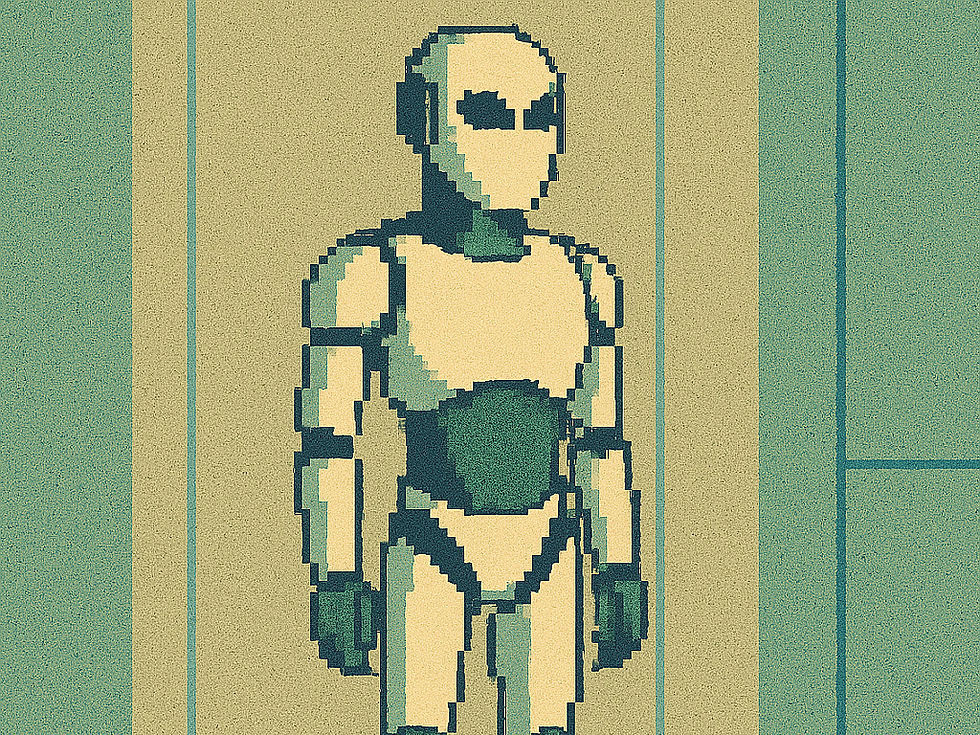
In the world of artificial intelligence (AI), a dataset serves as the foundation upon which models are constructed, trained, and refined. Simply put, a dataset is a structured collection of data points tailored to represent the problem an AI system aims to address. This could include images for visual recognition tasks, text for language processing, or numerical values for predictive modeling. Much like a chef relies on fresh, high-quality ingredients to craft a delicious meal, AI developers depend on well-curated datasets to fuel effective and reliable models. A poorly chosen or mishandled dataset can lead to inaccurate predictions, biased outcomes, or an inability to adapt to real-world challenges. This blog post explores what defines a dataset in AI and unpacks the qualities that distinguish a good dataset from a bad one, offering practical insights for your AI journey.
A dataset in AI is more than just a pile of data; it’s a carefully assembled resource that reflects the scope and nuances of the problem at hand. For instance, an AI designed to identify bird species might use a dataset of bird photographs, audio recordings of their calls, or even migration statistics. The dataset acts as the training ground where the AI learns patterns, makes predictions, and hones its abilities. Its significance cannot be overstated: without a robust dataset, even the most advanced algorithms falter. Understanding its role is the first step toward appreciating what separates a stellar dataset from a substandard one.
Characteristics of a Good Dataset
A good dataset isn’t just a random assortment of information; it embodies specific traits that empower AI models to perform at their best. Here are the hallmarks of excellence:
1. Relevance
The data must align directly with the problem being solved. If you’re training an AI to detect road signs, your dataset should feature images of stop signs, yield signs, and speed limits, not pictures of beaches or forests. Relevance ensures the AI focuses on the right signals.
2. Quality
High-quality data is clean, accurate, and consistent. For example, images should be sharp and well-lit, while text should be free of typos or garbled phrasing. A dataset riddled with errors, like blurry photos or misspellings, can confuse the model and weaken its output.
3. Diversity
Real-world scenarios are varied, and a good dataset mirrors that complexity. Consider an AI meant to recognize voices: it should include accents, pitches, and background noises from different environments, not just a single speaker in a quiet room. Diversity equips the AI to handle a broad range of situations.
4. Size
While bigger isn’t always better, a sufficiently large dataset provides the volume of examples needed for the AI to learn effectively. A model predicting stock prices, for instance, benefits from years of historical data rather than a mere month’s worth. However, balance is key—too much irrelevant data wastes resources.
5. Balance
A balanced dataset ensures fair representation across categories. In a spam email filter, if 90% of the emails are non-spam and only 10% are spam, the AI might lean toward labeling everything as non-spam. Equal or adjusted proportions prevent such biases.
6. Labeling
For supervised learning, where the AI relies on predefined answers, accurate labels are essential. A dataset of animal images must correctly tag “tiger” versus “lion.” Mistakes in labeling can mislead the model, undermining its precision.
What Makes a Dataset Bad?
A bad dataset falls short in one or more of these areas, jeopardizing the AI’s success. Here’s what to watch out for:
Lack of Relevance
Data unrelated to the task introduces noise. Training a medical diagnosis AI with images of furniture instead of X-rays is a recipe for failure.
Poor Quality
Inconsistent or flawed data—like grainy videos or corrupted files—distorts the learning process, leading to unreliable results.
Insufficient Diversity
A narrow dataset limits adaptability. An AI trained solely on sunny weather photos will struggle to identify objects in rain or fog.
Small Size
Too little data starves the model of learning opportunities, often causing it to overfit, meaning it memorizes the training set but flounders on new inputs.
Imbalance
Uneven class distribution skews predictions. A fraud detection system trained on mostly legitimate transactions might overlook rare but critical fraud cases.
Incorrect Labeling
Mislabels teach the AI the wrong lessons. If “apples” are tagged as “oranges,” the model’s understanding collapses.
The fallout from a bad dataset can be severe: diminished accuracy, entrenched biases, or even ethical concerns in fields like healthcare or law enforcement. A flawed foundation yields a shaky structure.
Practical Tips for Crafting or Selecting a Good Dataset
Building or choosing the right dataset requires strategy. Here’s how to get it right:
Clarify Your Goal: Define the problem precisely to guide data selection. Know what success looks like before you start.
Source Wisely: Tap into credible, authoritative sources to ensure data integrity.
Clean and Prepare: Scrub the data of errors and standardize it for consistency—think of it as prepping ingredients before cooking.
Embrace Variety: Seek out diverse examples and balance them to reflect reality fairly.
Label with Care: Use skilled annotators or reliable tools, and double-check for accuracy.
Leverage Resources: Explore existing datasets or enhance yours through augmentation, like rotating images to simulate new angles.
Conclusion
A dataset is the lifeblood of any AI project, and its quality can make or break your model’s performance. By focusing on relevance, quality, diversity, size, balance, and meticulous labeling, you lay the groundwork for AI that excels. A good dataset isn’t about amassing endless data—it’s about curating the right data for the job. Take the time to build or select wisely, and your AI will reward you with precision, adaptability, and trustworthiness. In the fast-evolving landscape of artificial intelligence, a solid dataset is your competitive edge.
—The LearnWithAI.com Team