How to Improve Memory in an AI System
- learnwith ai
- 5 days ago
- 3 min read
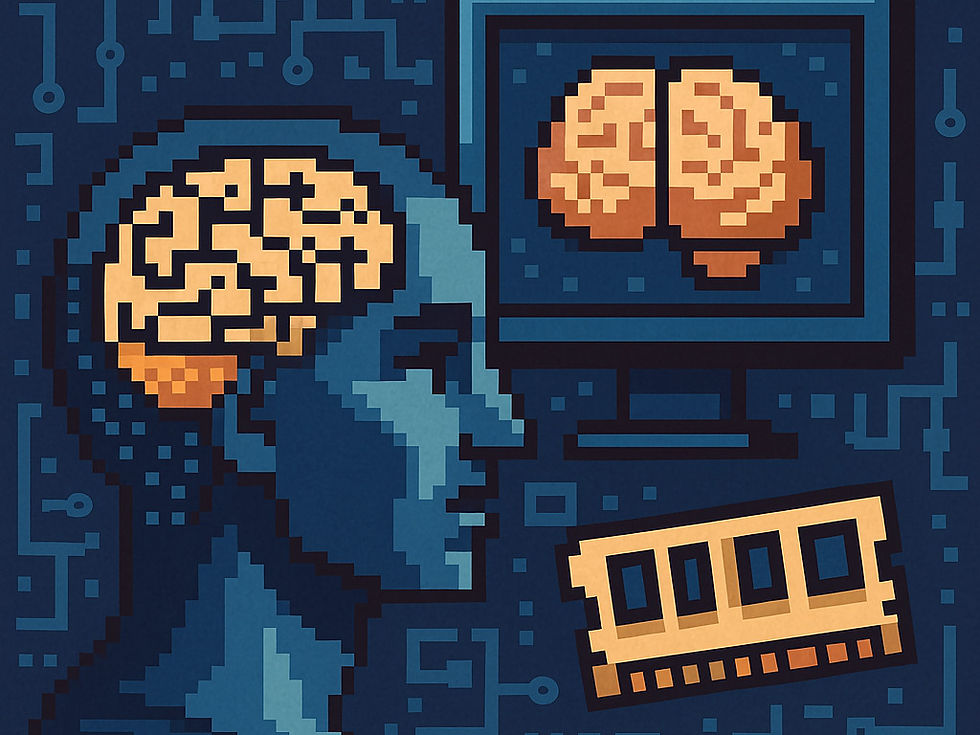
Sam Altman recently revealed that OpenAI is working on improving memory in AI systems, a development poised to radically enhance how AI models reason, interact, and evolve over time. But what does it mean to improve memory in artificial intelligence? Let’s dive into the technical and strategic implications of this advancement, and explore why it could be one of the most important breakthroughs in the field.
1. Why Memory Matters in AI
Traditional AI systems like GPT-4 process each user input independently, relying on context windows that are limited to recent interactions. While effective for many use cases, this approach lacks the ability to retain and recall past experiences, goals, or user preferences over longer periods.
Memory, in human terms, enables us to build relationships, adapt based on experience, and plan for the future. The same applies to AI. Introducing memory allows the system to:
Remember past conversations
Adjust behavior based on prior interactions
Learn continuously over time
Develop personalized outputs
2. From Stateless to Stateful Intelligence
Most current AI models operate as stateless systems they treat each prompt as isolated. A memory-augmented AI transforms this paradigm by becoming stateful, meaning it accumulates knowledge with use.
This transition involves the integration of:
Memory banks that store interaction histories
Retrieval mechanisms to access relevant past information
Updating protocols that adapt knowledge over time
Think of it as a fusion of short-term recall and long-term retention, forming a brain-like architecture.
3. How Memory Can Be Improved
Improving memory in AI involves several techniques, such as:
Vector Databases: Efficient ways to store and retrieve contextual embeddings
Reinforcement Learning from Human Feedback (RLHF): Aligning memory with human intent
Attention Mechanisms: Focusing on the most relevant stored data
External Memory Modules: Inspired by neuroscience and used in models like Neural Turing Machines
Memory Compression: Summarizing past experiences to save space and optimize retrieval
Each of these approaches contributes to a smarter, more context-aware AI that evolves with its environment.
4. Challenges Ahead
Improving AI memory isn't as simple as storing more data. It introduces new complexities:
Privacy Concerns: Persistent memory may retain sensitive user data
Bias Reinforcement: Remembering flawed outputs could perpetuate mistakes
Scalability Issues: Long-term memory systems require vast storage and efficient indexing
Control Mechanisms: Users should be able to manage, erase, or view what the AI remembers
The key lies in designing transparent, ethical, and user-controllable memory systems.
5. Real-World Applications
The benefits of advanced AI memory stretch across industries:
Healthcare: Track patient history and suggest contextual diagnostics
Education: Build personalized curriculums based on learning patterns
Finance: Detect anomalies over time, not just instant behavior
Productivity Tools: Remember user preferences, tone, and goals
Companionship and Coaching AI: Develop long-term relationships and understanding
6. What This Means for the Future
Improving memory in AI will shift models from reactive assistants to adaptive collaborators. It moves us closer to Artificial General Intelligence (AGI), where machines learn across time, reason across domains, and develop continuity of thought.
OpenAI’s focus on memory could lead to the emergence of AI that evolves not only with datasets but through lived digital experience. It’s not just about knowledge it’s about
wisdom built over time.
Final Thoughts
As Sam Altman hints at the future of AI, the message is clear: memory will become a core part of machine intelligence. Not only does it expand what AI can do it changes how AI thinks. We are witnessing the dawn of a new architecture where remembering becomes as crucial as computing.
—The LearnWithAI.com Team